Yageer: 5 years to build a data middle platform, saving 70% of daily effort.
Starting from a small garment factory in Ningbo, Zhejiang, the 45-year-old Youngor Group has maintained growth in scale and profits through digital concepts and business penetration. In 2018, it established the first intelligent factory in China's garment industry, achieving transparent and controllable production processes, and reducing the suit production cycle from 45 days to 32 days. A data dashboard displays real-time sales across the board, with the performance ranking of various marketing companies, and a clear comparison of revenue and sales across channels.
Like other clothing companies, Youngor has faced several major challenges in its development:
First, the multi-brand strategy and diverse channels generate massive amounts of data, accumulating over 300 million rows of data tables and more than 30TB of storage to date. If we calculate that one page has 10,000 characters (approximately 2KB), 30TB is equivalent to 150 million pages. At this scale of data, without value extraction and processing to support business growth, it is merely a cost. How to turn cost into value has become Youngor's first challenge.
Advertisement
Second, although the digital transformation started early, there are still pain points in fully leveraging data-driven efficiency. Around 2018, Youngor had completed the construction of basic business systems, but the data from various systems was not integrated. From design to the sales terminal, there are dozens of application systems based on business scenarios, which are dozens of independent data silos. To call data from these systems, one would need dozens of sets of usernames and passwords. From corporate decision-makers to store managers, without professional technology, it would take a lot of effort to find the needed data.
Youngor 5G Smart Factory
How to break the invisible data "department walls" and barriers between different systems, to gather streams into a river, turning data lakes into a "data ocean" for Youngor, has become the key to digital transformation. In 2018, Youngor proactively embraced the concept of a data mid-end platform and officially cooperated with Alibaba in 2019 to start building a data mid-end platform. Currently, the company has linked various business systems from fabric R&D, manufacturing, to smart marketing at the sales terminal based on the data mid-end platform. Through data construction and governance, it has provided decision-making basis and improved efficiency in business applications.
On April 24, 2024, at the first stop of the "Data Alumni Association" series of exchange meetings jointly held by Alibaba Lingyang and Tsinghua University's Data Governance and other research centers, Youngor Group CIO Wang Xin and Youngor Group Big Data Director Zhu Xianbo shared the company's digital intelligence transformation path and key achievements in data governance with the First Financial Business School.
I. Data Mid-end Platform: Cutting into Core Business Scenarios, Serving the Company's "Capillary Points" Decision-making
After proposing a five-year plan based on the data mid-end platform, Youngor mainly achieved four upgrades: platform upgrades based on big data technology and data governance, data asset completion, application scenario expansion, and interaction method simplification.
Taking application scenarios and interaction methods as examples, Youngor took the lead in cutting into core business scenarios (especially the front-end sales link), and has now launched around 190 application scenarios. By accumulating data insights, it helps all levels of the company make daily operational decisions. Wang Xin stated that these decisions are "small decisions," not the survival points of the enterprise, but "capillary points."From the daily decision-making of management to the scheduling of store sales assistants, the value of a data mid-end lies in "truly putting data to use," simplifying processes and improving efficiency in daily work, ultimately helping management make better decisions. Taking store decision-making and membership operations as examples:
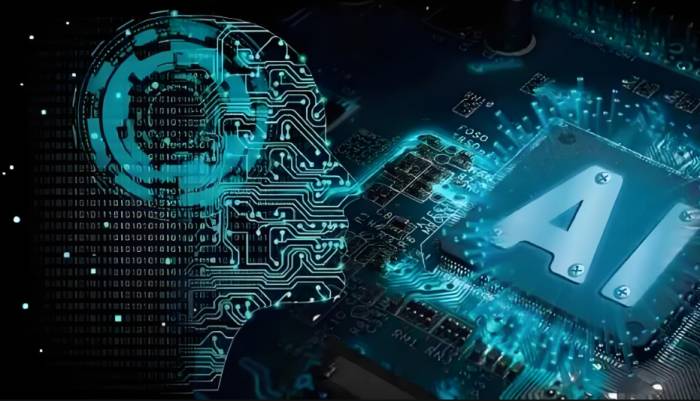
Store Panoramic Perspective: Assisting Management Decisions and Reducing Store Manager's Administrative Work
In the past, under a data environment where it was difficult to see the whole picture, management often needed to carry a stack of reports to visit stores before making decisions to understand the real store situation, such as store opening investment, decoration costs, personnel turnover, etc.; while store managers were under performance pressure and also needed to manage downward and report upward—these administrative tasks often took up a lot of time:
First, there was a need to manually enter and report sales situations; second, the data required for reporting was scattered across various business systems (for example, HR data was collected in the HR system, and logistics data was collected within the logistics system), making it time-consuming to search and requiring high data technology skills; third, the required data was affected by permissions, making it impossible to access the system.
After building a data mid-end, both the management and store sales assistants at Youngor have a more convenient way to obtain data. In its data portal, there are three data perspectives:
1. Divided by data characteristics. This is the data division method that most companies will adopt, collecting reports from various fields such as sales, logistics, finance, membership, audit, and manufacturing together.
2. Custom reports. Employees can freely drag and drop fields according to their needs.
3. Theme portals centered around the brand's various levels of perspective. Brand management can view their own sales, logistics, and other reports, and store managers also have a 360° panoramic view centered around the store. In 2023, Youngor also launched a large model intelligent BI application called Chat BI with the help of NLP technology, allowing employees to obtain data through search.
Youngor's brand HELLYHANSEN
A panoramic data centered on the store perspective not only facilitates management's understanding of the store situation but also reduces the daily basic administrative work of store managers by 60%-70%. In addition, when store managers arrange shifts for sales assistants, they can quickly determine through data whether the other party is more suitable for the day shift or the night shift, and whether they are more suitable for selling regular-priced products or discounted products—reducing the intervention of "people" can increase the correctness of decision-making, and also allows them to focus their energy on honing sales scripts and improving sales skills, ultimately enhancing the overall store performance.II. Data Governance: The Challenge of "Consensus is Hard to Achieve"
In the process of corporate digital transformation, technology is often not the biggest challenge. For instance, the governance of "data quality" is a case in point. Data itself does not deceive, but the difficulty lies in unifying data standards and the lack of standardized business processes, which can lead to data pollution, rendering it unable to provide practical reference value for business decision-making.
The complexity of Youngor's business also exacerbates the complexity and difficulty of data requirements and processing—even a single "revenue amount" can vary significantly due to factors such as mall deductions and financial tax deductions. If the data standards across different channels are not aligned, daily discrepancies of hundreds of thousands of yuan can arise.
Especially for the apparel industry, which places great emphasis on several key indicators, "four rates and two efficiencies": the "four rates" refer to the growth rate of sales, gross margin, cost-to-expense ratio, and recovery rate; the two efficiencies refer to labor efficiency and sales efficiency per square meter. For instance, sales efficiency per square meter (which refers to the average turnover per unit area of a store, i.e., sales divided by store area), different stores might report different figures such as the building area, operating area, or display area. Some stores might even underreport the area to achieve a higher sales efficiency. The intermingling of various interests and subjective intentions makes data governance the most challenging part of corporate data construction.
Therefore, the big data department has spent a significant amount of time integrating data on organization, products, personnel, etc., and has conducted a clear sorting of various dimensions and over 400 indicators. During this process, there are two key points:
1. Unifying data indicators.
2. Clarifying "interest boundaries" by standardizing business processes—that is, determining who should do what and to what extent.
When unifying data indicators, the big data department has sorted out key fields in each business process and provided detailed and clear data standards based on business needs: for example, "season" will be further divided into "Spring I," "Spring II," "Spring III," etc., according to business needs; and "store area" will also be updated in a timely manner with the renovation status. If there are doubts about the standards or criteria from the business department, the data indicator will be submitted to the Youngor Data Decision Committee (mainly composed of finance personnel) for judgment until a consensus is reached, and the final results will be publicly announced within the company. Since the establishment of the data platform, data indicators have been iterated on average every month, bringing data feedback closer to the needs of management demands and business applications.
Youngor Big Data Director, Zhu Xianbo
A more standardized indicator confirmation process can ensure data quality to a certain extent, leading to a fairer performance evaluation and assessment, or providing suggestions for decision-making directions. Zhu Xianbo said that after collecting several important indicators from the company's major regions, the data department will divide the indicators of each region into four quadrants, proposing corresponding suggestions for the current situation of the region: for example, if a region has a high gross margin but has room for improvement in recovery rate, turnover can be enhanced by optimizing promotional strategies.III. "To do a good job, one must first sharpen one's tools"
"Data mid-end is never a fleeting or impulsive project; it must be a long-term process," said Wang Xin. Yageer's plan for the next three to five years includes system integration as the theme, AI as the main thread, behavioral data for insight, and fragmented time for production.
In its three-layer data architecture of data sources, mid-end, and applications, Lingyang Dataphin and Quick BI play significant roles in the data mid-end layer and application layer, respectively, providing a safe and stable guarantee for the enterprise's current integration of omni-domain data and future planning.
At the data application layer, Yageer's flexible and multi-perspective data portal is being implemented based on Quick BI. As an analytical BI reporting tool, it meets the daily data acquisition needs at all levels of the enterprise, greatly reducing the threshold for employees to use data.
In the mid-end layer, Yageer has integrated a total of 16 systems, more than 900 tables, and over 400 sets of indicators through Dataphin. It is understood that Dataphin's main advantages are threefold:
1. It has the advantages of low-cost storage, high performance, and high stability.
2. It is one of the few full-link products on the market, more integrated than other data tools, capable of integrating data from different sources such as cloud and offline databases. Other tools often require third-party tools for integration.
3. The already diverse and complex enterprise data continues to expand in volume—while traditional data tools are mainly distributed in a single-machine manner, it is difficult to scale up with the changes in data volume. Dataphin adopts a distributed data processing method, which can flexibly scale up storage and computing power.
This means that enterprises no longer need to spend a lot of time on integration, greatly improving the efficiency of the data department in data retrieval. "Good tools save a lot of work effort. With the right tools, all our focus can be on business and data," said Zhu Xianbo.
Post Comment